Elżbieta Trypka
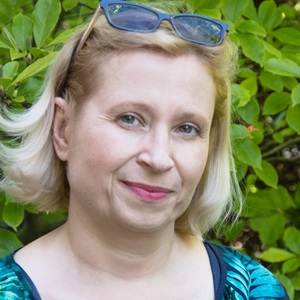
Title
Screening for early onset of dementia using voice as biomarker
Abstract
Introduction: We present a screening method for early dementia using features based on sound objects as voice biomarkers.Â
Methods: The final dataset used for machine learning models consisted of 266 observations, with a distribution of 186 healthy individuals, 46 diagnosed with Alzheimer’s, and 34 with MCI. This method is based on six-second recordings of the sustained vowel /a/ spoken by the subject. The main original contribution of this work is the use of carefully crafted features based on sound objects. This approach allows one to first represent the sound spectrum in a more accurate way than the standard spectrum, and then build interpretable features containing relevant information about subjects’ control over their voice.Â
Results: ROC AUC obtained in this work for distinguishing healthy subjects from those with MCI was 0.85, while accuracy was 0.76. For distinguishing between healthy subjects and those with either MCI or Alzheimer’s the results were 0.84, 0.77, respectively.Â
Conclusion: The use of features based on sound objects enables screening for early dementia even on very short recordings of language-independent voice samples.Â
Biography
Elżbieta Trypka, MD, PhD, is an Assistant Professor at the Department of Psychiatry at the Wroclaw Medical University. She is a specialist in psychiatry, a co-founder, and a board member of the Polish Psychogeriatric Society.
Her main research interests focus on neurodegenerative diseases of the central nervous system, early diagnostic possibilities, and existing biochemical disorders in the course of Alzheimer’s disease. She is the author and co-author of scientific papers published in Polish and international journals. Notably she serves as the participant and leader of the many projects related to research seeking early biomarkers of dementia disorders. She leads pioneering research on Sound Objects is Vivid Mind’s unique, patented approachto audio decomposition, separation and analysis using sound vectoring methods and algorithms. This advanced signal processing methodology is based on a sinusoidal model that offers a radically new approach to computational analysis of the auditory scene.